Figure5
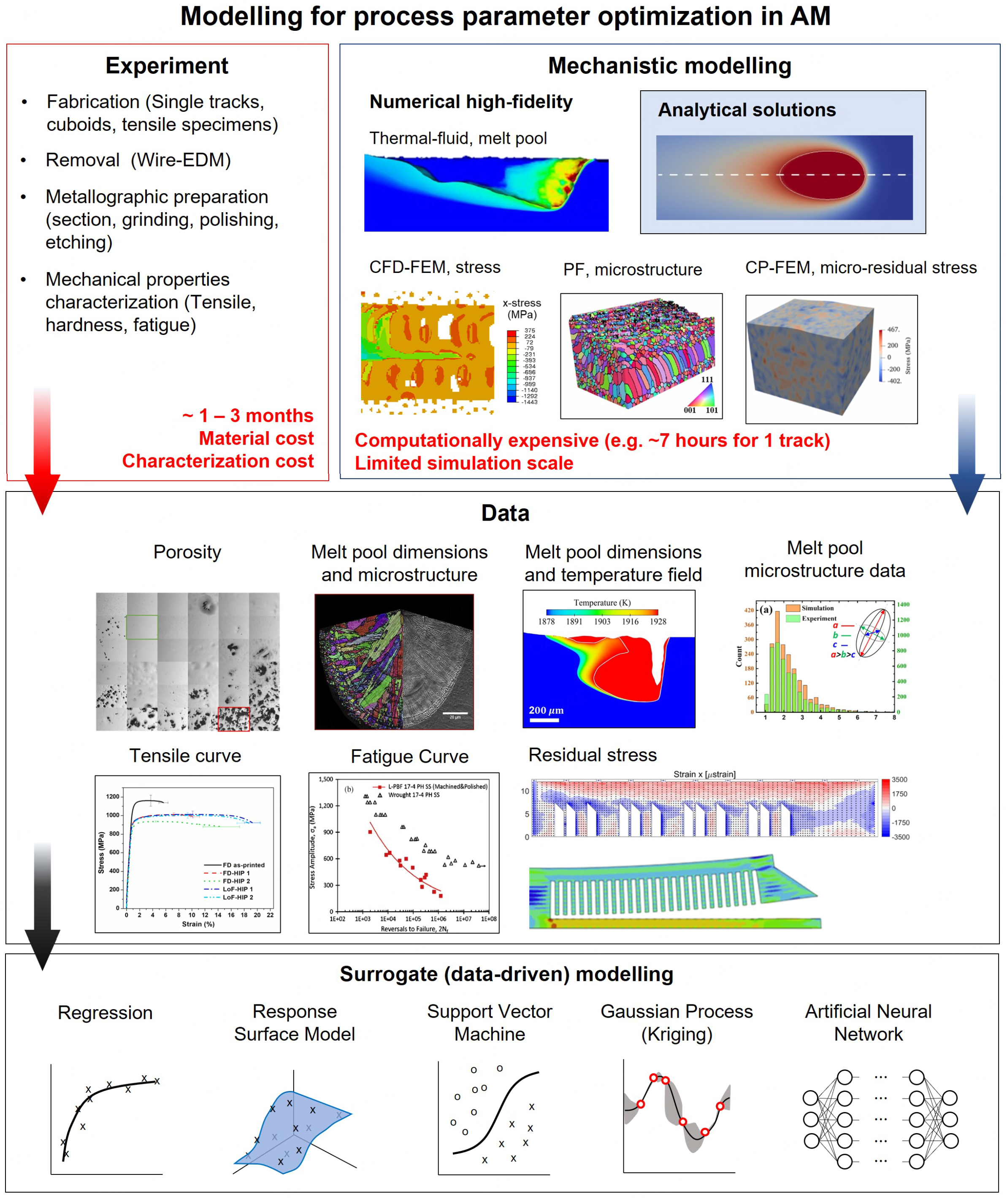
Figure 5. Modelling for optimization in AM. The process map in AM has mostly been characterized using experiments, which are costly. Analytical models (e.g. Rosenthal's solution [152]) provide rapid prediction of the process map but become inaccurate when convection effects are significant. High-fidelity models (e.g. thermal-fluid melt pool simulations for melt pool [153], novel computational fluid dynamics and finite element model (CFD-DEM) coupling for stress prediction [23], phase field (PF) models for microstructure [154] and crystal plasticity and finite element model coupling (CP-FEM) for micro-residual stresses [155]) can account for such complex physics but have limited simulation scale. Data generated from experiments include porosity [96], melt pool dimensions and microstructure [89], tensile characteristics [96] and fatigue characteristics [156]. Similarly, simulations can generate data such as melt pool dimensions and the temperature field [35], melt pool microstructure data [154] and residual stresses [157, 158]. The data generated by these models can be further analyzed with data-driven algorithms to generate surrogate models to generate the response maps.