fig6
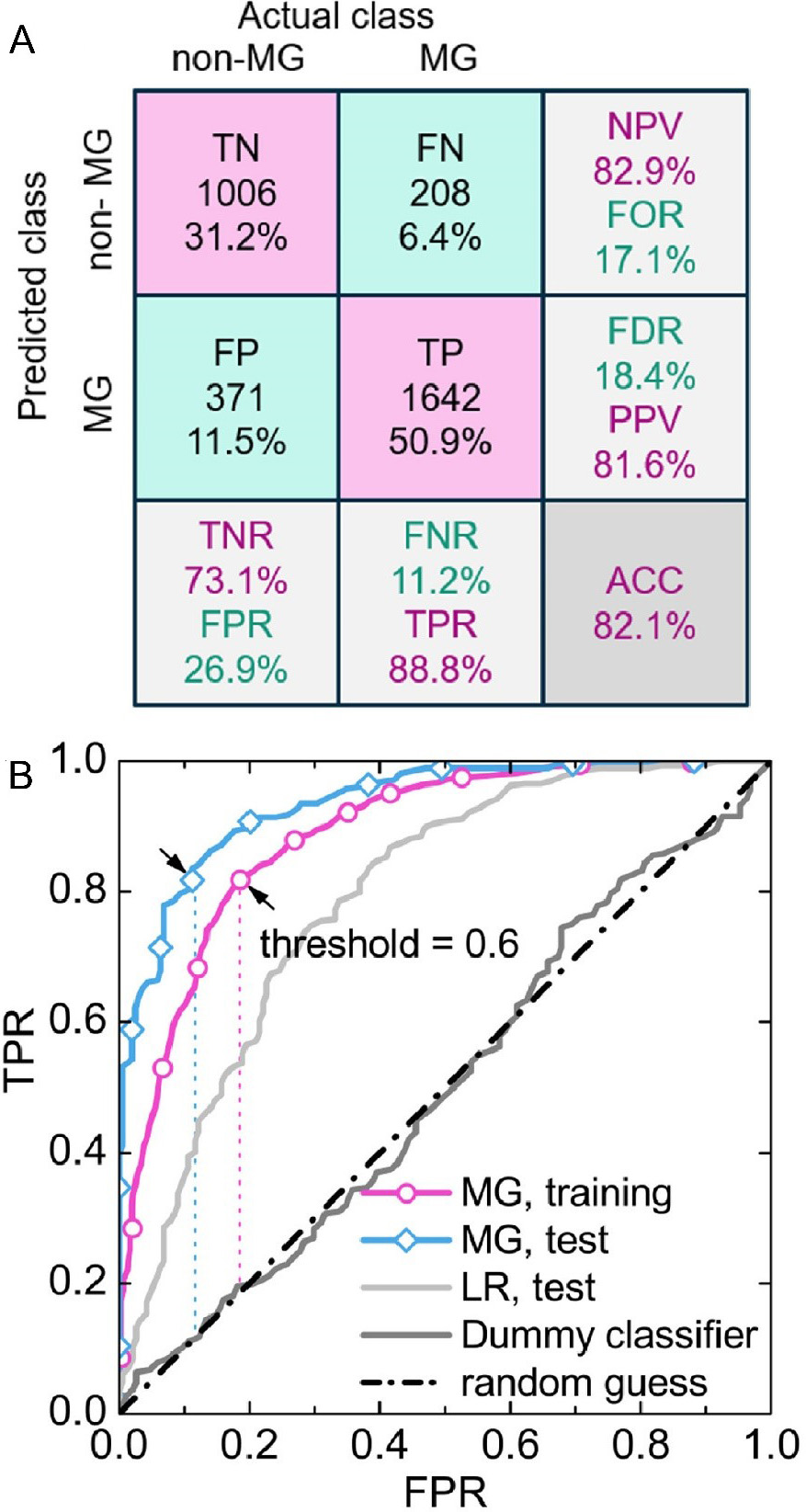
Figure 6. (A) Confusion matrix and (B) ROC curves for a classification ML model for MG formation. Note that the terminology and derivations in (A) are true negative (TN), false negative (FN), false positive (FP), true positive (TP), negative predictive value (NPV = TN/(TN + FN), false omission rate [FOR = FN/(TN + FN)], false discovery rate [FDR = FP/(FP + TP)], positive predictive value [PPV = TP/(TP + FP)], true negative rate [TNR = TN/(TN + FP)], false positive rate [FPR = FP/(FP + TN)], false negative rate [FNR = FN(TP + FN)], true positive rate [TPR = TP/(TP + FN)] and accuracy [ACC = (TP+TN)/(TP + TN + FP + FN)]. Reprinted from Ref.[24], copyright (2020), with permission from Elsevier. ROC: Receiver operating characteristic; ML: machine learning; MG: metallic glass.