fig4
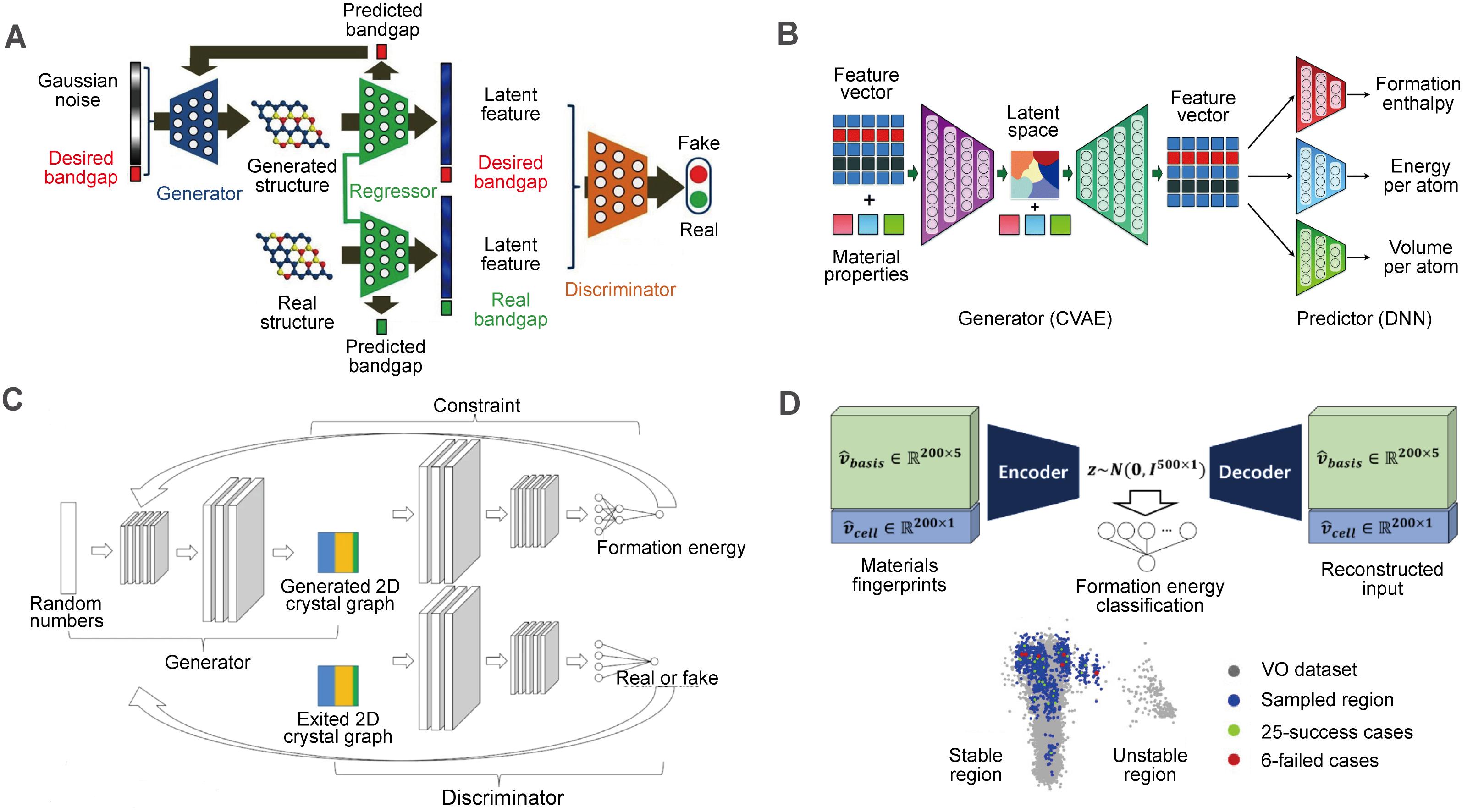
Figure 4. Networks for inverse design of inorganic solid materials. (A) Regressional and conditional GAN (RCGAN) framework. This figure is quoted with permission from Dong et al.[81]. (B) Deep learning based inorganic material generator (DING) framework consisting of a generator module and a predictor module. This figure is quoted with permission from Pathak et al.[51]. (C) Constrained crystals deep convolutional GAN (CCDCGAN) framework. This figure is quoted with permission from Long et al.[21]. (D) Materials generator module in iMatGen and the visualization of the latent space. This figure is quoted with permission from Noh et al.[54].